Invited Talks
Modern NLP runs on Transformers. Large language models are possible because of system successes in making Transformers bigger, faster, and longer-range. However, 5 years after the advent of BERT and GPT, it is still an open question whether the central routing component of Transformers, Self-Attention, is central to their success in pretraining, or whether it is worth developing large-scale systems for alternative approaches. Inspired by an off-hand wager on this topic https://www.isattentionallyouneed.com, this talk will be an overview of recent work exploring the use of alternative approaches for routing in large-scale NLP architectures. After giving background on the best practices and context of modern NLP, I will describe alternative approaches, primarily focusing on static methods based on state-space models (SSMs) and long-range convolutions. I will conclude by discussing the current empirical results and theoretical properties of these models, as well as paths for their future systems development as competitive technologies.
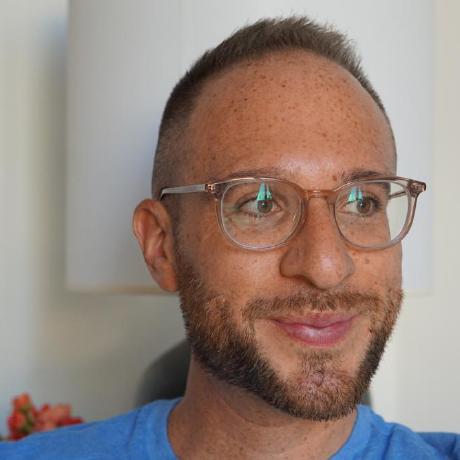
Alexander Rush
Alexander "Sasha" Rush is an Associate Professor at Cornell Tech and a researcher at Hugging Face. His current research interests are the intersection of natural language processing and deep generative modeling with applications in text generation, efficient inference, and controllability. In addition to academic research, he has written several popular open-source software projects supporting NLP research, data science, and virtual academic conferences such as NeurIPS and ACL. His research and open-source projects have received paper and demo awards at major NLP, visualization, and hardware conferences, an NSF Career Award, and a Sloan Fellowship. He tweets and blogs, mostly about coding and ML, at @srush_nlp.
Large language models are fluent text generators, but they struggle at generating factual, correct content, even when paired with tools such as information retrieval and agent programming frameworks. In this talk, I’ll discuss Demonstrate-Search-Predict (DSP), a system we are developing at Stanford to let users build highly accurate applications using LLMs and external tools. DSP offers a declarative programming model, where users write an application using control flow in Python and calls to ML components such as an LLM or a neural information retrieval system. Given such an application and a small amount of data, DSP systematically improves the application by tuning the ML components to get high quality results, by automatically generating better prompts for each model involved, fine-tuning models, etc. We show that with even a few tens of examples, DSP can match state-of-the-art solutions on multiple knowledge-intensive tasks, and that it can then systematically improve both task performance and computational efficiency without requiring manual tuning or prompt engineering from a developer. We also discuss and compare with other emerging approaches to turn LLMs into reliable software components.
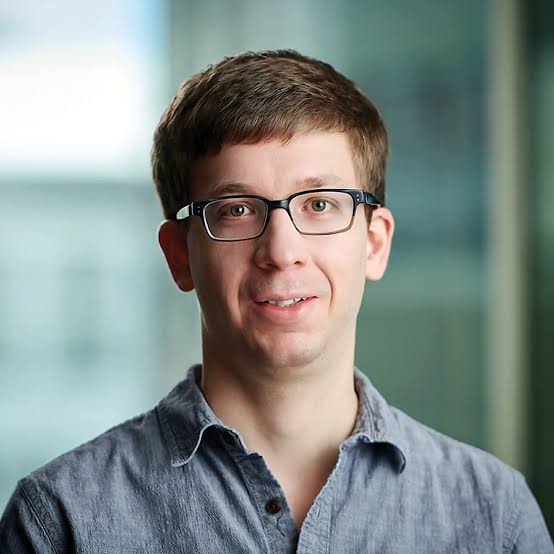
Matei Zaharia
Matei Zaharia is an Associate Professor of Computer Science at Stanford (moving to UC Berkeley later this year) and Chief Technologist and Cofounder of Databricks. His research has spanned distributed systems, databases, security and machine learning, with the most recent focus on systems for machine learning, natural language processing, and information retrieval. Matei started and contributed to multiple widely used open source projects including Apache Spark (his PhD project at UC Berkeley), MLflow, Dolly, Delta Lake, and ColBERT. His research was recognized through the 2014 ACM Doctoral Dissertation Award, an NSF CAREER Award, and the US Presidential Early Career Award for Scientists and Engineers (PECASE).