Registration Desk: Registration Check-in Desk Mon 29 Aug 07:00 a.m.
Oral: Outstanding Papers Mon 29 Aug 08:45 a.m.
[ Exhibit Hall A ]
We present the design of a new large scale orchestration layer for accelerators. Our system, Pathways, is explicitly designed to enable exploration of new systems and ML research ideas, while retaining state of the art performance for current models. Pathways uses a sharded dataflow graph of asynchronous operators that consume and produce futures, and efficiently gang-schedules heterogeneous parallel computations on thousands of accelerators while coordinating data transfers over their dedicated interconnects. Pathways makes use of a novel asynchronous distributed dataflow design that lets the control plane execute in parallel despite dependencies in the data plane. This design, with careful engineering, allows Pathways to adopt a single-controller model that makes it easier to express complex new parallelism patterns. We demonstrate that Pathways can achieve performance parity (~100% accelerator utilization) with state-of-the-art systems when running SPMD computations over 2048 TPUs, while also delivering throughput comparable to the SPMD case for Transformer models that are pipelined across 16 stages, or sharded across two islands of accelerators connected over a data center network.
[ Exhibit Hall A ]
The significant success of Deep Neural Networks (DNNs) is highly promoted by the multiple sophisticated DNN libraries. On the contrary, although some work have proved that Quadratic Deep Neuron Networks (QDNNs) show better non-linearity and learning capability than the traditional first-order DNNs, their neuron design suffers certain drawbacks from theoretical performance to practical deployment. In this paper, we first proposed a new QDNN neuron architecture design, and further developed QuadraLib, a QDNN library to provide architecture optimization and design exploration for QDNNs. Extensive experiments show that our design has better performance regarding prediction accuracy and computation consumption on multiple learning tasks.
[ Exhibit Hall A ]
[ Exhibit Hall A ]
Benefited from expanding cloud infrastructure, today's neural networks have increasingly high performance trained on the cloud. Model researchers spent months of sweat competing for an extra few percent of model accuracy. However, when these models are actually deployed on edge devices in practice, very often, the performance is dropping over 10% all of a sudden without obvious reasons. The key challenge is that there is not much visibility to ML inference execution on edge devices, and very little awareness of potential issues during the edge deployment process. ML-EXray provides visibility into layer-level details of the ML execution, helps developers analyze and debug cloud-to-edge deployment issues. More often than not, the reason does not only lie in the model itself, but every operation throughout the data flow and the deployment process. Evaluations show that ML-EXray can effectively catch deployment issues, such as pre-processing bugs, quantization issues, suboptimal kernels; using ML-EXray, users need to write less than 15 line of code to fully examine the edge deployment pipeline; eradicating these issues, ML-EXray can correct model performance by up to 30%, pinpoint error-prune layers, guide users to optimize kernel execution latency by two orders of magnitude. Code and APIs will be released as …
[ Exhibit Hall A ]
High-performance primitives for mathematical operations on sparse vectors must deal with the challenges of skewed degree distributions and limits on memory consumption that are typically not issues in dense operations. We demonstrate that a sparse semiring primitive can be flexible enough to support a wide range of critical distance measures while maintaining performance and memory efficiency on the GPU. We further show that this primitive is a foundational component for enabling many neighborhood-based information retrieval and machine learning algorithms to accept sparse input. To our knowledge, this is the first work aiming to unify the computation of several critical distance measures on the GPU under a single flexible design paradigm and we hope that it provides a good baseline for future research in this area. Our implementation is fully open source and publicly available as part of the RAFT library of GPU-accelerated machine learning primitives (https://github.com/rapidsai/raft).
Invited Talk: Kunle Olukotun
Systems for ML and ML for Systems: A Virtuous Cycle
This talk is about the virtuous interplay between machine learning (ML) and systems. I will show examples of how systems optimized for ML computation can be used to train more accurate and capable ML models and how these ML models can be used to improve upon the ad-hoc heuristics used in system design and management. These improved systems can then be used to train better ML models. The latest trend in ML is the development of Foundation models. Foundation models are large pretrained models that have obtained state-of-the-art quality in natural language processing, vision, speech, and other areas. These models are challenging to train and serve because they are characterized by billions of parameters, irregular data access (sparsity) and irregular control flow. I will explain how Reconfigurable Dataflow Accelerators (RDAs) can be designed to accelerate foundation models with these characteristics. SambaNova Systems is using RDA technology to achieve record-setting performance on foundation models. I will describe how the RDAs can also be used to build Taurus, an intelligent network data plane that enables ML models to be used to manage computer networks at full line-rate bandwidths. In particular, a Taurus prototype detects two orders of magnitude more events in a security application than a state-of-the-art system based on conventional network technology.
Bio :The Future of MLSys: Industry and Academia - panel Mon 29 Aug 11:30 a.m.
Are you a budding researcher or engineer interested in MLSys or just curious about opportunities across industry and academia? Join our panel, “The Future of MLSys: Industry and Academia”, where representatives from industry and academia share their experiences in machine learning and systems. The panel will cover topics such as differences between MLSys research in industry vs. academia, the role of industry and academia in the field more broadly, and how you can get involved!
Signup here
Tutorial: Hasan Genc
Gemmini: Enabling Systematic Deep-Learning Architecture Evaluation via Full-Stack Integration
Please register online at this link if you would like to attend: https://forms.gle/Pd9tviuBHBno7G7F7
We present a tutorial that teaches users how to perform full-system, full-stack DNN accelerator evaluation using the Gemmini platform. Gemmini allows users to evaluate how a DNN hardware accelerator interacts with external components, like the cache hierarchy or virtual address translation scheme, to affect performance across the hardware-software-system stack.
With Gemmini, users can generate a variety of different DNN hardware accelerators, with different underlying system, SoC, and programming stack components. Users can evaluate the performance of their hardware accelerators on end-to-end workloads in a real-world system context, exposing how different system components, like the cache hierarchy, virtual address translation scheme, or operating system, impact performance in subtle but noticeable ways. Gemmini also allows users to program their applications at different “levels” of the programming stack, from high-level model compilation to low-level direct machine configuration. Overall, Gemmini enables users to explore and evaluate a variety of different DNN accelerator and system configurations, exposing how these different parameters interact to impact end-to-end performance and efficiency.
Gemmini has been presented previously at DAC 2021, where it won the Best Paper award, as
well as at an IISWC 2021 tutorial.
Tutorial: Patel Dhaval
Time Series Anomaly Detection : Tools, Techniques & Tricks
This tutorial presents a design and implementation of a scikit-compatible system for detecting anomalies from time series data for the purpose of offering a broad range of algorithms to the end user, with special focus on unsupervised/semi-supervised learning. Given an input time series, we discuss how data scientist can construct four categories of anomaly pipelines followed by an enrichment module that helps to label anomaly. The tutorial provides an hand-on-experience using a deployed system on IBM API Hub for developer communities that aim to support a wide range of execution engines to meet the diverse need of anomaly workloads such as Serveless for CPU intensive work, GPU for deep-learning model training, etc.
Bio :Oral: Distributed and Parallel Learning Mon 29 Aug 01:00 p.m.
[ Exhibit Hall A ]
Graph Convolutional Networks (GCNs) have emerged as the state-of-the-art method for graph-based learning tasks. However, training GCNs at scale is still challenging, hindering both the exploration of more sophisticated GCN architectures and their applications to real-world large graphs. While it might be natural to consider graph partition and distributed training for tackling this challenge, this direction has only been slightly scratched the surface in the previous works due to the limitations of existing designs. In this work, we first analyze why distributed GCN training is ineffective and identify the underlying cause to be the excessive number of boundary nodes of each partitioned subgraph, which easily explodes the memory and communication costs for GCN training. Furthermore, we propose a simple yet effective method dubbed BNS-GCN that adopts random Boundary-Node-Sampling to enable efficient and scalable distributed GCN training. Experiments and ablation studies consistently validate the effectiveness of BNS-GCN, e.g., boosting the throughput by up to 16.2× and reducing the memory usage by up to 58%, while maintaining a full-graph accuracy. Furthermore, both theoretical and empirical analysis show that BNS-GCN enjoys a better convergence than existing sampling-based methods. We believe that our BNS-GCN has opened up a new paradigm for enabling GCN training …
[ Exhibit Hall A ]
We present the Sequential Aggregation and Rematerialization (SAR) scheme for distributed full-batch training of Graph Neural Networks (GNNs) on large graphs. Large-scale training of GNNs has recently been dominated by sampling-based methods and methods based on non-learnable message passing. SAR on the other hand is a distributed technique that can train any GNN type directly on an entire large graph. The key innovation in SAR is the distributed sequential rematerialization scheme which sequentially re-constructs then frees pieces of the prohibitively large GNN computational graph during the backward pass. This results in excellent memory scaling behavior where the memory consumption per worker goes down linearly with the number of workers, even for densely connected graphs. Using SAR, we report the largest applications of full-batch GNN training to-date, and demonstrate large memory savings as the number of workers increases. We also present a general technique based on kernel fusion and attention-matrix rematerialization to optimize both the runtime and memory efficiency of attention-based models. We show that, coupled with SAR, our optimized attention kernels lead to significant speedups and memory savings in attention-based GNNs.
[ Exhibit Hall A ]
[ Exhibit Hall A ]
Secure model aggregation is a key component of federated learning (FL) that aims at protecting the privacy of each user’s individual model while allowing for their global aggregation. It can be applied to any aggregation-based FL approach for training a global or personalized model. Model aggregation needs to also be resilient against likely user dropouts in FL systems, making its design substantially more complex. State-of-the-art secure aggregation protocols rely on secret sharing of the random-seeds used for mask generations at the users to enable the reconstruction and cancellation of those belonging to the dropped users. The complexity of such approaches, however, grows substantially with the number of dropped users. We propose a new approach, named LightSecAgg, to overcome this bottleneck by changing the design from random-seed reconstruction of the dropped users'' to
one-shot aggregate-mask reconstruction of the active users via mask encoding/decoding''. We show that LightSecAgg achieves the same privacy and dropout-resiliency guarantees as the state-of-the-art protocols while significantly reducing the overhead for resiliency against dropped users. We also demonstrate that, unlike existing schemes, LightSecAgg can be applied to secure aggregation in the asynchronous FL setting. Furthermore, we provide a modular system design and optimized on-device parallelization for scalable implementation, …
Oral: ML Compilers & Runtime Mon 29 Aug 02:15 p.m.
[ Exhibit Hall A ]
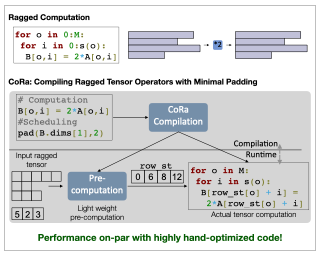
There is often variation in the shape and size of input data used for deep learning. In many cases, such data can be represented using tensors with non-uniform shapes, or ragged tensors. Due to limited and non-portable support for efficient execution on ragged tensors, current deep learning frameworks generally use techniques such as padding and masking to make the data shapes uniform and then offload the computations to optimized kernels for dense tensor algebra. Such techniques can, however, lead to a lot of wasted computation and therefore, a loss in performance. This paper presents CoRa, a tensor compiler that allows users to easily generate efficient code for ragged tensor operators targeting a wide range of CPUs and GPUs. Evaluating CoRa on a variety of operators on ragged tensors as well as on an encoder layer of the transformer model, we find that CoRa (i) performs competitively with hand-optimized implementations of the operators and the transformer encoder and (ii) achieves a 1.6 geomean speedup over PyTorch for the encoder on an Nvidia GPU and a 1.37 geomean speedup over TensorFlow for the multi-head attention module used in transformers on a 64-core ARM CPU.
[ Exhibit Hall A ]
We study fusion for deep neural networks (DNNs) in a just-in-time (JIT) compilation framework Apollo. It considers both memory- and compute-bound tensor operators for fusion, and integrates graph-level node grouping and operator-level loop fusion closely, widening the fusion search space. Apollo enables the upward feedback from the downstream loop optimizer, enforcing the graph engine to regenerate partition patterns amenable to the downstream pass and thus resolving the scalability issue. Besides data locality, Apollo also exploits the parallelism between independent tensor operators, further improving the performance of DNN workloads. Experimental results on training workloads show that Apollo outperforms TensorFlow and XLA by 1.86× and 1.37× on a single GPU, and 1.96× and 1.18× on multiple GPUs. Apollo also improves the performance of a vendor-provided DNN framework by 19.7% on a domain-specific accelerator. In addition, the results of inference workloads demonstrate the general applicability of our fusion framework.
[ Exhibit Hall A ]
Achieving high performance for compute-intensive operators in machine learning (ML) workloads is a crucial but challenging task. Many ML and system practitioners rely on vendor libraries or auto-schedulers to do the job. While the former requires large engineering efforts, the latter only supports static-shape workloads in existing works. It is difficult, if not impractical, to apply existing auto-schedulers directly to dynamic-shape workloads, as this leads to extremely long auto-scheduling time.We observe that the key challenge faced by existing auto-schedulers when handling a dynamic-shape workload is that they cannot construct a unified search space for all the possible shapes of the workload, because their search space is shape-dependent. To address this, we propose DietCode, a new auto-scheduler framework that efficiently supports dynamic-shape workloads by constructing a shape-generic search space and cost model. Under this construction, all shapes jointly search within the same space and update the same cost model when auto-scheduling, which is therefore more efficient compared with existing auto-schedulers.We evaluate DietCode using state-of-the-art machine learning workloads on a modern GPU. Our evaluation shows that DietCode has the following key strengths when auto-scheduling an entire model end-to-end: (1) reduces the auto-scheduling time by up to 5.88x less than the state-of-the-art auto-scheduler …
[ Exhibit Hall A ]
Applications of neural networks on edge systems have proliferated in recent years but the ever increasing model size makes neural networks not able to deploy on resource-constrained microcontrollers efficiently. We propose bit-serial weight pools, an end-to-end framework that includes network compression and acceleration of arbitrary sub-byte precision. The framework can achieve up to 8x compression compared to 8-bit networks by sharing a pool of weights across the entire network. We further propose a bit-serial lookup based software implementation that allows runtime-bitwidth tradeoff and is able to achieve more than 2.8x speedup and 7.5x storage compression compared to 8-bit networks, with less than 1% accuracy drop.
Oral: Systems for ML 1 Mon 29 Aug 04:00 p.m.
[ Exhibit Hall A ]
This paper presents the first industry-standard open-source machine learning (ML) benchmark to allow performance and accuracy evaluation of mobile devices with different AI chips and software stacks. The benchmark draws from the expertise of leading mobile-SoC vendors, ML-framework providers, and model producers. It comprises a suite of models that operate with standard data sets, quality metrics and run rules. We describe the design and implementation of this domain-specific ML benchmark. The current benchmark version comes as a mobile app for different computer vision and natural language processing tasks. The benchmark also supports non-smartphone devices, such as laptops and mobile PCs. Benchmark results from the first two rounds reveal the overwhelming complexity of the underlying mobile ML system stack, emphasizing the need for transparency in mobile ML performance analysis. The results also show that the strides being made all through the ML stack improve performance. Within six months, offline throughput improved by 3x, while latency reduced by as much as 12x. ML is an evolving field with changing use cases, models, data sets and quality targets. MLPerf Mobile will evolve and serve as an open-source community framework to guide research and innovation for mobile AI.
[ Exhibit Hall A ]
Deep learning on point clouds has received increased attention thanks to its wide applications in AR/VR and autonomous driving. These applications require low latency and high accuracy to provide real-time user experience and ensure user safety. Unlike conventional dense workloads, the sparse and irregular nature of point clouds poses severe challenges to running sparse CNNs efficiently on the general-purpose hardware, and existing sparse acceleration techniques for 2D images do not translate to 3D point clouds. In this paper, we introduce TorchSparse, a high-performance point cloud inference engine that accelerates the sparse convolution computation on GPUs. TorchSparse directly optimizes the two bottlenecks of sparse convolution: irregular computation and data movement. It adopts adaptive MM grouping to trade computation for better regularity, achieving 1.4-1.5x speedup for matrix multiplication. It also optimizes the data movement by adopting vectorized, quantized and fused locality-aware memory access, reducing the memory movement cost by 2.7x. Evaluated on seven representative models across three benchmark datasets, TorchSparse achieves 1.6x and 1.5x measured end-to-end speedup over the state-of-the-art MinkowskiEngine and SpConv, respectively.
[ Exhibit Hall A ]
Deep Neural Networks (DNNs) are often trained in parallel on a cluster of virtual machines (VMs) so as to reduce training time. However, this requires explicit cluster management, which is cumbersome and often results in costly overprovisioning of resources. Training DNNs on serverless compute is an attractive alternative that is receiving growing interest. In a serverless environment, users do not need to handle cluster management and can scale compute resources at a fine-grained level while paying for resources only when actively used. Despite these potential benefits, existing serverless systems for DNN training are ineffective because they are limited to CPU-based training and bottlenecked by expensive distributed communication. We present Hydrozoa, a system that trains DNNs on serverless containers with a hybrid-parallel architecture that flexibly combines data- and model-parallelism. Hydrozoa supports GPU-based training and leverages hybrid-parallelism and serverless resource scaling to achieve up to 155.5x and 5.4x higher throughput-per-dollar compared to existing serverless and VM-based training systems. Hydrozoa also allows users to implement dynamic worker-scaling policies during training. We show that dynamic worker scaling improves statistical training efficiency and reduces training costs.
[ Exhibit Hall A ]
Input pipelines, which ingest and transform input data, are an essential part of training Machine Learning (ML) models. However, it is challenging to implement efficient input pipelines, as it requires reasoning about parallelism, asynchrony, and variability in fine-grained profiling information. Our analysis of over two million ML jobs in Google datacenters reveals that a significant fraction of model training jobs could benefit from faster input data pipelines. At the same time, our analysis indicates that most jobs do not saturate host hardware, pointing in the direction of software-based bottlenecks. Motivated by these findings, we propose Plumber, a tool for finding bottlenecks in ML input pipelines. Plumber uses an extensible and interpretable operational analysis analytical model to automatically tune parallelism, prefetching, and caching under host resource constraints. Across five representative ML pipelines, Plumber obtains speedups of up to 47x for misconfigured pipelines. By automating caching, Plumber obtains end-to-end speedups of over 50% compared to state-of-the-art tuners.
[ Exhibit Hall A ]
Graph neural networks (GNNs) are a new class of powerful machine learning models, but easy programming and efficient computing is often at odds. Current GNN frameworks are based on a message passing paradigm, and allow the concise expression of GNN models using built-in primitives and user defined functions (UDFs). While built-in primitives offer high performance, they are limited in expressiveness; UDFs are flexible, but often have low performance and use excessive memory. In this paper, we propose Graphiler, a compiler stack for GNNs which achieves high performance while offering the flexibility of the UDF programming interface. At the core of Graphiler is a novel abstraction called Message Passing Data Flow Graph (MP-DFG), which enables optimizations that substantially reduce computational redundancy and memory footprint, and optimizes both homogeneous and heterogeneous GNNs under a unified framework. Experiments show Graphiler can accelerate UDF GNNs by up to two orders of magnitude, and achieve performance close to or superior to expert implementations, and do so with substantial memory savings.
Welcome Reception Mon 29 Aug 05:30 p.m.
The Opening Reception will be held on Monday evening in the Mission City Ballroom at 5:30pm.