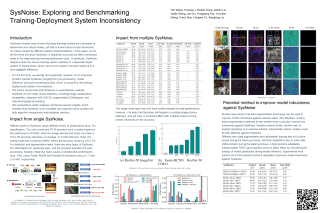
Abstract
Extensive studies have shown that deep learning models are vulnerable to adversarial and natural noises, yet little is known about model robustness on noises caused by different system implementations. In this paper, we for the first time introduce SysNoise, a frequently occurred but often overlooked noise in the deep learning training-deployment cycle. In particular, SysNoise happens when the source training system switches to a disparate target system in deployments, where various tiny system mismatch adds up to a non-negligible difference. We first identify and classify SysNoise into three categories based on the inference stage; we then build a holistic benchmark to quantitatively measure the impact of SysNoise on 20+ models, comprehending image classification, object detection, instance segmentation and natural language processing tasks. Our extensive experiments revealed that SysNoise could bring certain impacts on model robustness across different tasks and common mitigations like data augmentation and adversarial training show limited effects on it. Together, our findings open a new research topic and we hope this work will raise research attention to deep learning deployment systems accounting for model performance.
Abstract
Sparsely-gated mixture-of-experts (MoE) has been widely adopted to scale deep learning models to trillion-plus parameters with fixed computational cost. The algorithmic performance of MoE relies on its token routing mechanism that forwards each input token to the right sub-models or experts. While token routing dynamically determines the amount of expert workload at runtime, existing systems suffer inefficient computation due to their static execution, namely static parallelism and pipelining, which does not adapt to the dynamic workload.We present Tutel, a highly scalable stack design and implementation for MoE with dynamically adaptive parallelism and pipelining. Tutel designs an identical layout for distributing MoE model parameters and input data, which can be leveraged by switchable parallelism and dynamic pipelining methods without mathematical inequivalence or tensor migration overhead. This enables adaptive parallelism/pipelining optimization at zero cost during runtime. Based on this key design, Tutel also implements various MoE acceleration techniques including Flexible All-to-All, two-dimensional hierarchical (2DH) All-to-All, fast encode/decode, etc. Aggregating all techniques, Tutel finally delivers 4.96x and 5.75x speedup of a single MoE layer over 16 and 2,048 A100 GPUs, respectively, over the previous state-of-the-art.Our evaluation shows that Tutel efficiently and effectively runs a real-world MoE-based model named SwinV2-MoE, built upon Swin Transformer …
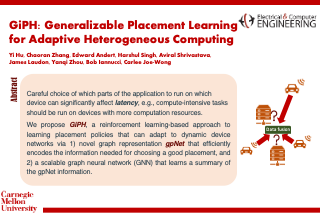
Abstract
Careful placement of a distributed computational application within a target device cluster is critical for achieving low application completion time. The problem is challenging due to its NP-hardness and combinatorial nature. In recent years, learning-based approaches have been proposed to learn a placement policy that can be applied to unseen applications, motivated by the problem of placing a neural network across cloud servers. These approaches, however, generally assume the device cluster is fixed, which is not the case in mobile or edge computing settings, where heterogeneous devices move in and out of range for a particular application. To address the challenge of scaling to different-sized device clusters and adapting to the addition of new devices, we propose a new learning approach called GiPH, which learns policies that generalize to dynamic device clusters via a novel graph representation gpNet that efficiently encodes the information needed for choosing a good placement, and a scalable graph neural network (GNN) that learns a summary of the gpNet information. GiPH turns the placement problem into that of finding a sequence of placement improvements, learning a policy for selecting this sequence that scales to problems of arbitrary size. We evaluate GiPH with a wide range of …
Abstract
Training and inference with graph neural networks (GNNs) on massive graphs in a distributed environment has been actively studied since the inception of GNNs, owing to the widespread use and success of GNNs in applications such as recommendation systems and financial forensics. This paper is concerned with minibatch training and inference with GNNs in distributed settings, where the necessary partitioning of vertex features across distributed storage causes feature communication to become a major bottleneck that hampers scalability.To significantly reduce the communication volume without compromising prediction accuracy, we propose a policy for caching data associated with frequently accessed vertices in remote partitions. The proposed policy is based on an analysis of vertex-wise inclusion probabilities (VIP) during multi-hop neighborhood sampling, which may expand the neighborhood far beyond the partition boundary of the graph. The VIP analysis not only enables the elimination of the communication bottleneck, but also offers a means to organize in-memory data by prioritizing GPU storage for the most frequently accessed vertex features. We present SALIENT++, which extends the prior state-of-the-art SALIENT system to work with partitioned feature data and leverages the VIP-driven caching policy. SALIENT++ retains the local training efficiency and scalability of SALIENT by using a deep pipeline …
Abstract
Deep neural networks are often highly over-parameterized, and weight pruning or sparsification can be an effective method for reducing both their memory footprints and inference latencies. Among existing pruning strategies, unstructured or fine-grained pruning typically achieves the highest compression ratios and lowest task errors; unfortunately, such irregular and non-uniform sparsity leads to significant load imbalance and consequently degraded performance on parallel architectures. Recent attempts to accelerate unstructured sparsity on GPUs have focused on the 90-99% sparsity regime, where most modern DNNs have been shown to lose considerable accuracy. In this paper, we introduce the uniform sparsity pattern that ensures a constant number of non-zero values per row of the sparse matrix, and thus lends itself well to efficient, load-balanced execution on modern parallel architectures. Uniform sparsity achieves useful speedups in both the moderate (50-90%) and high (90%+) sparsity regimes and performs similarly to unstructured sparsity in terms of accuracy. We describe how uniform sparsity is induced on DNN weights and present optimized kernels that accelerate uniform sparsity on GPUs. We evaluate uniform sparsity on a range of real-world networks and synthetic data, and demonstrate mean performance improvements of up to 62% over the NVIDIA cuSparse library at iso-accuracy settings.
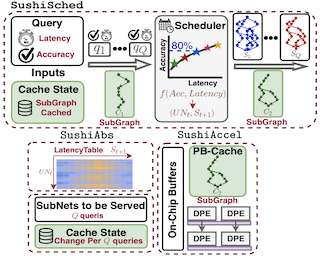
Abstract
A growing number of applications depend on Machine Learning (ML) functionality and benefits from both higher quality ML predictions and better timeliness (latency) at the same time. A growing body of research in computer architecture, ML, and systems software literature focuses on reaching better latency/accuracy tradeoffs for ML models. Efforts include compression, quantization, pruning, early-exit models, mixed DNN precision, as well as ML inference accelerator designs that minimize latency and energy, while preserving delivered accuracy. All of them, however, yield improvements for a single static point in the latency/accuracy tradeoff space. We make a case for applications that operate in dynamically changing deployment scenarios, where no single static point is optimal. We draw on a recently proposed weight-shared SuperNet mechanism to enable serving a stream of queries that uses (activates) different SubNets within this weight-shared construct. This creates an opportunity to exploit the inherent temporal locality with our proposed SubGraph Stationary (SGS) optimization. We take a hardware-software co-design approach with a real implementation of SGS in SushiAccel and the implementation of a software scheduler SushiSched controlling which SubNets to serve and what to cache in real-time. Combined, they are vertically integrated into SUSHI—an inference serving stack. For the stream of …
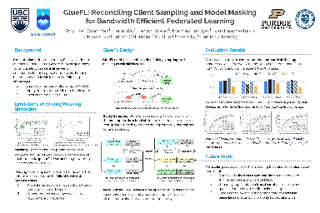
Abstract
Federated learning (FL) is an effective technique to directly involve edge devices in machine learning training while preserving client privacy. However, the substantial communication overhead of FL makes training challenging when edge devices have limited network bandwidth. Existing work to optimize FL bandwidth overlooks downstream transmission and does not account for FL client sampling. In this paper we propose GlueFL, a framework that incorporates new client sampling and model compression algorithms to mitigate low download bandwidths of FL clients. GlueFL prioritizes recently used clients and bounds the number of changed positions in compression masks in each round. Across three popular FL datasets and three state-of-the-art strategies, GlueFL reduces downstream client bandwidth by 27% on average and reduces training time by 29% on average.
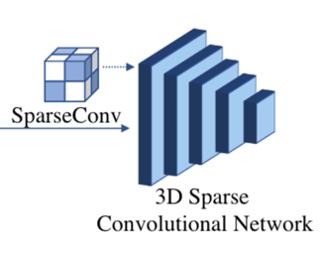
Abstract
Sparse convolution is the key operator in widely-used 3D point cloud networks. However, due to the high sparsity of voxelized input point cloud data, three main challenges need to be solved for efficient sparse convolution in current 3D point cloud engines: (1) Memory under-utilization: the mapping information from input data to weight parameters of 3D point cloud networks is sparse, leading to up to 79.97% redundant memory access and under-utilized memory space; (2) Computation under-utilization: previous FGMS (Fused Gather-Matrix-Multiplication-Scatter) operations in sparse convolution are executed sequentially, leading to a GPU computation utilization of only 22.84%; (3) Input dynamics: a single and static dataflow in the current point cloud engines cannot always achieve the best performance on different input point cloud data.To tackle these challenges, we propose PCEngine, an efficient sparse convolution engine for voxel-based 3D point cloud networks. PCEngine proposes a novel coded-CSR (Compress Sparse Row) format to represent the mapping information without redundancy. PCEngine also introduces the indicator-assisted segmented FGMS fusion scheme to fully utilize the computation resources on GPU hardware. PCEngine further deploys a heuristic adaptive dataflow for input dynamics. Extensive experimental results show that, PCEngine achieves 1.81× and 1.64× speedup on average for sparse convolution operation and …
Abstract
While the quality of machine learning services largely relies on the volume of training data, data regulations such as the General Data Protection Regulation (GDPR) impose stringent requirements on data transfer. Federated learning has emerged as a popular approach for enabling collaborative machine learning without sharing raw data. To facilitate the rapid development of federated learning, efficient and user-friendly federated learning systems are essential. Despite many existing federated learning systems designed for deep learning, tree-based federated learning systems have not been well exploited. This paper presents a tree-based federated learning system under a histogram-sharing scheme, named FedTree, that supports both horizontal and vertical federated training of GBDTs with configurable privacy protection techniques. Our extensive experiments show that FedTree achieves competitive accuracy to centralized training while incurring much less computational cost than the other generic federated learning systems.
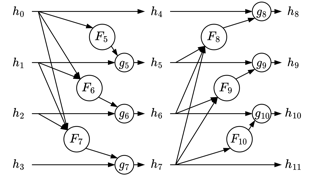
Abstract
This work introduces RevSilo, the first reversible bidirectional multi-scale feature fusion module. Like other reversible methods, RevSilo eliminates the need to store hidden activations by recomputing them. However, existing reversible methods do not apply to multi-scale feature fusion and are, therefore, not applicable to a large class of networks. Bidirectional multi-scale feature fusion promotes local and global coherence and has become a de facto design principle for networks targeting spatially sensitive tasks, e.g., HRNet (Sun et al., 2019a) and EfficientDet (Tan et al., 2020). These networks achieve state-of-the-art results across various computer vision tasks when paired with high-resolution inputs. However, training them requires substantial accelerator memory for saving large, multi-resolution activations. These memory requirements inherently cap the size of neural networks, limiting improvements that come from scale. Operating across resolution scales, RevSilo alleviates these issues. Stacking RevSilos, we create RevBiFPN, a fully reversible bidirectional feature pyramid network. RevBiFPN is competitive with networks such as EfficientNet while using up to 19.8x lesser training memory for image classification. When fine-tuned on MS COCO, RevBiFPN provides up to a 2.5% boost in AP over HRNet using fewer MACs and a 2.4x reduction in training-time memory.
Abstract
We study a novel and important communication pattern in large-scale model-parallel deep learning (DL), which we call cross-mesh resharding. This pattern emerges when the two paradigms of model parallelism – intra-operator and inter-operator parallelism – are combined to support large models on large clusters. In cross-mesh resharding, a sharded tensor needs to be sent from a source device mesh to a destination device mesh, on which the tensor may be distributed with the same or different layouts. We formalize this as a many-to-many multicast communication problem, and show that existing approaches either are sub-optimal or do not generalize to different network topologies or tensor layouts, which result from different model architectures and parallelism strategies. We then propose two contributions to address cross-mesh resharding: an efficient broadcast-based communication system, and an “overlapping-friendly" pipeline schedule. On microbenchmarks, our overall system outperforms existing ones by up to 10x across various tensor and mesh layouts. On end-to-end training of two large models, GPT-3 and U-Transformer, we improve throughput by 10% and 50%, respectively.
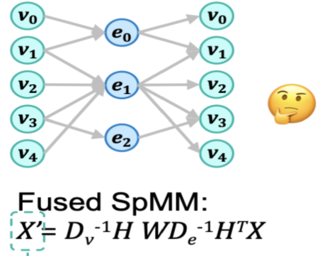
Abstract
Hypergraph Neural Network (HyperGNN) is an emerging type of Graph Neural Networks (GNNs) that can utilize hyperedges to model high-order relationships among vertices. Current GNN frameworks fail to fuse two message-passing steps from vertices to hyperedges and hyperedges to vertices, leading to high latency and redundant memory consumption. The following challenges need to be solved for efficient fusion in HyperGNNs: (1) Inefficient partition: hardware-efficient and workload-balanced partitions are required for parallel workers to process two consecutive message passing steps after fusion. (2) Workload-Agnostic Format: current data formats like Compressed Sparse Row (CSR) fail to represent a two-step computation workload. (3) Heavy writing conflicts: partitioning leads to heavy writing conflicts when updating the same vertex.To enable efficient fusion for HyperGNNs, we present HyperGef. HyperGef proposes an edge-split workload balance partition scheme to achieve higher efficiency and better workload balancing. To represent the workload after fusion and partition, HyperGef introduces a novel fusion workload-aware format. HyperGef also introduces a shared memory-aware grouping scheme to reduce writing conflicts. Extensive experiments demonstrate that our fused kernel outperforms the NVIDIA cuSPARSE kernel by 3.31x. By enabling efficient fusion for HyperGNNs, HyperGef achieves 2.25x to 3.99x end-to-end speedup on various HyperGNN models compared with state-of-the-art frameworks …
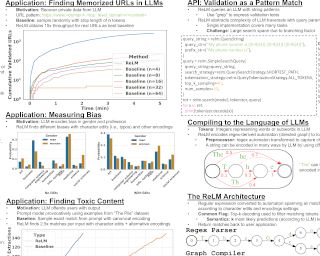
Abstract
Although large language models (LLMs) have been touted for their ability to generate natural-sounding text, there are growing concerns around possible negative effects of LLMs such as data memorization, bias, and inappropriate language. Unfortunately, the complexity and generation capacities of LLMs make validating (and correcting) such concerns difficult. In this work, we introduce ReLM, a system for validating and querying LLMs using standard regular expressions. ReLM formalizes and enables a broad range of language model evaluations, reducing complex evaluation rules to simple regular expression queries. Our results exploring queries surrounding memorization, gender bias, toxicity, and language understanding show that ReLM achieves up to 15× higher system efficiency, 2.5× data efficiency, and increased statistical and prompt-tuning coverage compared to state-of-the-art ad-hoc queries. ReLM offers a competitive and general baseline for the increasingly important problem of LLM validation.
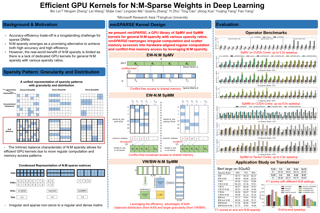
Abstract
N:M sparsity is becoming increasingly popular due to its promise to achieve both high model accuracy and computational efficiency for deep learning. However, the real-world benefit of N:M sparsity is limited as there is a lack of dedicated GPU kernel implementations for general N:M sparsity with various sparsity ratios. In this work, we present nmSPARSE, a library of efficient GPU kernels for two fundamental operations in neural networks with N:M sparse weights: sparse matrix-vector multiplication (SpMV) and sparse matrix-matrix multiplication (SpMM). By leveraging the intrinsic balance characteristic of N:M sparsity, nmSPARSE kernels rearrange irregular computation and scattered memory accesses in sparse matrix multiplication into hardware-aligned regular computation and conflict-free memory accesses at runtime. Evaluated on NVIDIA A100 GPU, nmSPARSE kernels achieve up to 5.2× speedup on SpMV and 6.0× speedup on SpMM over the fastest baseline. End-to-end studies on transformer models demonstrate that using nmSPARSE outperforms other baselines.
Abstract
Gradient checkpointing is an optimization that reduces the memory footprint by re-computing some operations instead of saving their activations. Previous works on checkpointing have viewed this as a tradeoff between peak memory and performance. However, we argue that this framing does not account for a key aspect of modern deep learning systems -- operator fusion. In this work, we demonstrate that with a fusion aware checkpointing algorithm, we can transcend the runtime-memory tradeoffs of traditional checkpointing and improve both memory and runtime simultaneously. We evaluate our algorithm on a wide range of standard neural network models as well as some novel patterns. We achieve a geomean of 12% throughput improvement over an existing compiled baseline, and the maximum batch size that can be attained is up to 1.75 times larger on standard models. In novel patterns, we achieve up to a 10x improvement, with by a 5x reduction in peak memory.
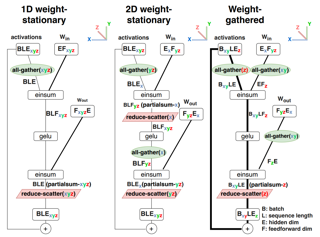
Abstract
We study the problem of efficient generative inference for Transformer models, in one of its most challenging settings: large deep models, with tight latency targets and long sequence lengths. Better understanding of the engineering tradeoffs for inference for large Transformer-based models is important as use cases of these models are growing rapidly throughout application areas. We develop a simple analytical model for inference efficiency to select the best multi-dimensional partitioning techniques optimized for TPU v4 slices based on the application requirements. We combine these with a suite of low-level optimizations to achieve a new Pareto frontier on the latency and model FLOPS utilization (MFU) tradeoffs on 500B+ parameter models that outperforms theFasterTransformer suite of benchmarks. We further show that with appropriate partitioning, the lower memory requirements of multiquery attention (i.e. multiple query heads share single key/value head) enables scaling up to32×larger context lengths. Finally, we achieve a low-batch-size latency of 29ms per token during generation (using int8 weight quantization) and a 76% MFU during large-batch-size processing of input tokens, while supporting a long 2048-token context length on the PaLM 540B parameter model.
Abstract
Profiling is a standard practice used to investigate the efficiency of software and hardware operation at runtime and is a crucial part of proving new concepts, debugging problems, and optimizing performance. However, most machine learning (ML) developers find profiling secondary to their goal of improving model accuracy or just too difficult (especially with existing ML tools). As a result, profiling is frequently an afterthought, and so many ML developers rely on opaque metrics such as iteration time and GPU utilization which give little insight into why ML training may be slow. This leads developers to spend excessive time investigating performance issues. In this work, we aim to provide better tools to the large group of ML developers who currently do not profile their deep neural network (DNN) training workloads or are not happy with existing tools. To help ML developers investigate and understand time-use in DNN training, we propose Hotline, a novel profiler designed specifically for runtime bottleneck identification. Hotline is the first profiler to automatically annotate a standard data format for program runtime traces with DNN concepts that most ML developers are familiar with, i.e. the DNN training loop and model architecture. Hotline does so without modifying DNN libraries …
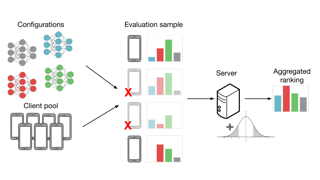
Abstract
Hyperparameter tuning is critical to the success of federated learning applications. Unfortunately, appropriately selecting hyperparameters is challenging in federated networks, as issues of scale, privacy, and heterogeneity introduce noise in the tuning process and make it difficult to faithfully evaluate the performance of various hyperparameters. In this work we perform the first systematic study on the effect of noisy evaluation in federated hyperparameter tuning. We first identify and rigorously explore key sources of noise, including client subsampling, data and systems heterogeneity, and data privacy. Surprisingly, our results indicate that even small amounts of noise can have a significant impact on tuning methods—reducing the performance of state-of-the-art approaches to that of naive baselines. To address noisy evaluation in such scenarios, we propose a simple and effective approach that leverages public proxy data to boost evaluation signal. Our work establishes general challenges, baselines, and best practices for future work in federated hyperparameter tuning.
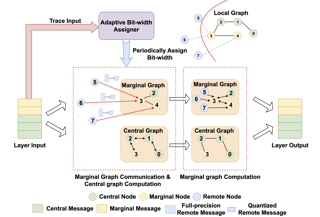
Abstract
Distributed full-graph training of Graph Neural Networks (GNNs) over large graphs is bandwidth-demanding and time-consuming. Frequent exchanges of node features, embeddings and embedding gradients (all referred to as messages) across devices bring significant communication overhead for nodes with remote neighbors on other devices (marginal nodes) and unnecessary waiting time for nodes without remote neighbors (central nodes) in the training graph. This paper proposes an efficient GNN training system, AdaQP, to expedite distributed full-graph GNN training. We stochastically quantize messages transferred across devices to lower-precision integers for communication traffic reduction and advocate communication-computation parallelization between marginal nodes and central nodes. We provide theoretical analysis to prove fast training convergence (at the rate of O(T^{-1}) with T being the total number of training epochs) and design an adaptive quantization bit-width assignment scheme for each message based on the analysis, targeting a good trade-off between training convergence and efficiency. Extensive experiments on mainstream graph datasets show that AdaQP substantially improves distributed full-graph training's throughput (up to 3.01 X) with negligible accuracy drop (at most 0.30%) or even accuracy improvement (up to 0.19%) in most cases, showing significant advantages over the state-of-the-art works.
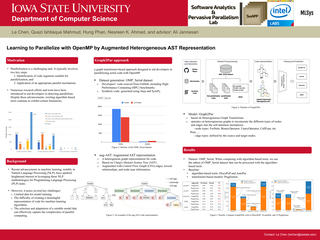
Abstract
Detecting parallelizable code regions is a challenging task, even for experienced developers. Numerous recent studies have explored the use of machine learning for code analysis and program synthesis, including parallelization, in light of the success of machine learning in natural language processing. However, applying machine learning techniques to parallelism detection presents several challenges, such as the lack of an adequate dataset for training, an effective code representation with rich information, and a suitable machine learning model to learn the latent features of code for diverse analyses. To address these challenges, we propose a novel graph-based learning approach called Graph2Par that utilizes a heterogeneous augmented abstract syntax tree (Augmented-AST) representation for code. The proposed approach primarily focused on loop-level parallelization with OpenMP. Moreover, we create an OMP_Serial dataset with 18598 parallelizable and 13972 non-parallelizable loops to train the machine learning models. Our results show that our proposed approach achieves the accuracy of parallelizable code region detection with 85\% accuracy and outperforms the state-of-the-art token-based machine learning approach. These results indicate that our approach is competitive with state-of-the-art tools and capable of handling loops with complex structures that other tools may overlook.
Abstract
To deploy compute-intensive neural networks on resource-constrained edge systems, developers use model optimization techniques that reduce model size and computational cost. Existing optimization tools are application-agnostic -- they optimize model parameters solely in view of the neural network accuracy -- and can thus miss optimization opportunities. We propose ApproxCaliper, the first programmable framework for application-aware neural network optimization. By incorporating application-specific goals, ApproxCaliper facilitates more aggressive optimization of the neural networks compared to application-agnostic techniques. We perform experiments on five different neural networks used in two real-world robotics systems: a commercial agriculture robot and a simulation of an autonomous electric cart. Compared to Learning Rate Rewinding (LRR), a state-of-the-art structured pruning tool used in an application-agnostic setting, ApproxCaliper achieves 5.3x higher speedup and 2.9x lower GPU resource utilization, and 36x and 6.1x additional model size reduction, respectively.
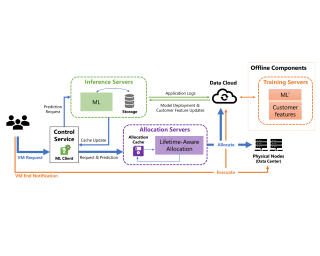
Abstract
The emergence of machine learning technology has motivated the use of ML-based predictors in computer systems to improve their efficiency and robustness. However, there are still numerous algorithmic and systems challenges in effectively utilizing ML models in large-scale resource management services that require high throughput and response latency of milliseconds. In this paper, we describe the design and implementation of a VM allocation service that uses ML predictions of the VM lifetime to improve packing efficiencies. We design lifetime-aware placement algorithms that are provably robust to prediction errors and demonstrate their merits in extensive real-trace simulations. We significantly upgraded the VM allocation infrastructure of Microsoft Azure to support such algorithms that require ML inference in the critical path. A robust version of our algorithms has been recently deployed in production, and obtains efficiency improvements expected from simulations.
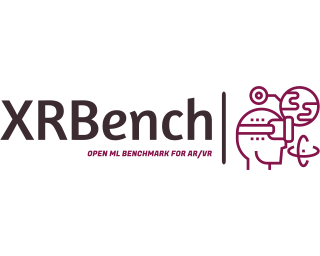
Abstract
Real-time multi-task multi-model (MTMM) workloads, a new form of deep learning inference workloads, are emerging for applications areas like extended reality (XR) to support metaverse use cases. These workloads combine user interactivity with computationally complex machine learning (ML) activities. Compared to standard ML applications, these ML workloads present unique difficulties and constraints. Real-time MTMM workloads impose heterogeneity and concurrency requirements on future ML systems and devices, necessitating the development of new capabilities. This paper begins with a discussion of the various characteristics of these real-time MTMM ML workloads and presents an ontology for evaluating the performance of future ML hardware for XR systems. Next, we present XRBENCH, a collection of MTMM ML tasks, models, and usage scenarios that execute these models in three representative ways: cascaded, concurrent, and cascaded-concurrency for XR use cases. Finally, we emphasize the need for new metrics that capture the requirements properly. We hope that our work will stimulate research and lead to the development of a new generation of ML systems for XR use cases. XRBench is available as an open-source project: https://github.com/XRBench
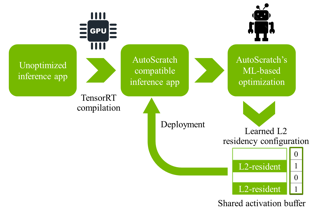
Abstract
Taking advantage of the L2 residency control mechanism introduced with NVIDIA's Ampere GPUs, we propose a Machine Learning (ML) based framework called AutoScratch to automatically discover and optimize the L2 residency for inference-oriented GPUs, effectively removing any human involvement from the optimization loop. AutoScratch bridges the gap between the performance of an explicitly controlled scratchpad memory and the convenience of a hardware-controlled cache. We develop two versions of AutoScratch, AutoScratch-RL harnessing reinforcement learning (RL) and AutoScratch-EA leveraging a state-of-the-art evolutionary algorithm (EA). We integrate AutoScratch with NVIDIA's TensorRT framework to fully automate the optimization pipeline for arbitrary DL inference applications. We evaluate AutoScratch on NVIDIA's L4 GPU silicon using MLPerf inference workloads and show that AutoScratch reduces off-chip DRAM traffic by 29% and improves the overall performance by 9% (up to 22%).
Abstract
This paper introduces a Unified Convolution Framework (UCF) that incorporates various existing sparse convolutions in a unified abstraction. This work is in contrast to the common library-based approach that requires much engineering effort because each different sparse convolution must be implemented separately. Instead, it employs a tensor compiler approach that can flexibly explore convolutions with various program transformations; however, no compiler can currently support various sparse convolutions flexibly to our knowledge. In particular, the Tensor Algebra Compiler (TACO) can support a variety of sparse formats but cannot declare convolutions because a tensor cannot be accessed by a linear combination of index variables. We extend TACO's Einsum language to support an affine index expression to declare a convolution. Our method is also compatible with TACO's format and scheduling language, enabling various sparse convolution implementations to be explored. Our experimental results demonstrate that TACO-UCF achieves 1.32× and 8.3× average speedups on a filter sparse convolution and a submanifold sparse convolution, respectively, over state-of-the-art libraries on CPU. TACO-UCF on GPU outperforms the state-of-the-art GPU library on filter sparse convolution of ResNet50 by an average of 1.47× at 80% sparsity. We also demonstrate TACO-UCF outperforms on a neighbor retrieval of a submanifold sparse convolution …
Abstract
Edge Impulse is a cloud-based machine learning operations (MLOps) platform for developing embedded and edge ML (TinyML) systems that can be deployed to a wide range of hardware targets. Current TinyML workflows are plagued by fragmented software stacks and heterogeneous deployment hardware, making ML model optimizations difficult and unportable. We present Edge Impulse, a practical MLOps platform for developing TinyML systems at scale. Edge Impulse addresses these challenges and streamlines the TinyML design cycle by supporting various software and hardware optimizations to create an extensible and portable software stack for a multitude of embedded systems. As of Oct. 2022, Edge Impulse hosts 118,185 projects from 50,953 developers.
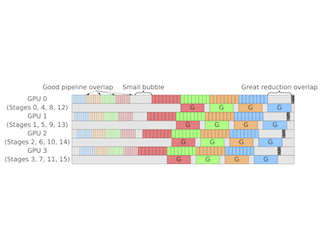
Abstract
We introduce Breadth-First Pipeline Parallelism, a novel training schedule which optimizes the combination of pipeline and data parallelism. Breadth-First Pipeline Parallelism lowers training time, cost and memory usage by combining a high GPU utilization with a small batch size per GPU, and by making use of fully sharded data parallelism. Experimentally, we observed an increase of up to 43% in training throughput for a 52 billion-parameter model using a small batch size per GPU compared to Megatron-LM, which would reduce the training time and cost by the same amount on a large GPU cluster.
Abstract
Parallel training is mandatory in order to maintain performance efficiency and tackle memory constraints for deep neural network (DNN) models. For this purpose, a critical optimization in order to tune a parallelism strategy is to schedule tensors onto device memory in compilation time. In this paper, we present a safe and optimized solver for this problem capturing a general parallel scenario to enable execution in open-source MindSpore framework. The input is a computational graph and a partition of its operators into streams of execution, which may run in parallel. First, we design algorithms to efficiently and provably decide if it is safe, for any two tensors, to reuse memory. Second, given such a set of reuse constraints, as well as a set of contiguous constraints to enable bulk communication among processing elements, we design algorithms to assign an offset to each tensor, such that all constraints are satisfied and total memory is minimized. Our experiments in parallel training of a variety of DNNs demonstrate nearly optimal, improved in some cases, memory consumption compared to state-of-the-art (adapted for our setting) and a sequential execution lower bound. Our algorithms show compilation time gains of up to 44% in determining safety and up …
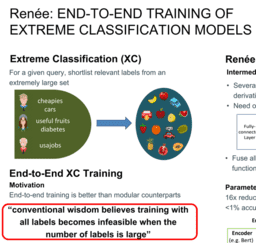
Abstract
The goal of Extreme Multi-label Classification (XC) is to learn representations that enable mapping input texts to the most relevant subset of labels selected from an extremely large label set, potentially in hundreds of millions. Given the extreme scale, conventional wisdom believes it is infeasible to train an XC model in an end-to-end manner. Thus, for training efficiency, several modular and sampling-based approaches to XC training have been proposed in the literature. In this paper, we identify challenges in the end-to-end training of XC models and devise novel optimizations that improve training speed over an order of magnitude, making end-to-end XC model training practical. Furthermore, we show that our end-to-end trained model, Renee, delivers state-of-the-art accuracy in a wide variety of XC benchmark datasets. Renee code will be released publicly.
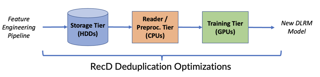
Abstract
We present RecD (Recommendation Deduplication), a suite of end-to-end infrastructure optimizations across the Deep Learning Recommendation Model (DLRM) training pipeline. RecD addresses immense storage, preprocessing, and training overheads caused by feature duplication inherent in industry-scale DLRM training datasets. Feature duplication arises because DLRM datasets are generated from interactions. While each user session can generate multiple training samples, many features’ values do not change across these samples. We demonstrate how RecD exploits this property, end-to-end, across a deployed training pipeline. RecD optimizes data generation pipelines to decrease dataset storage and preprocessing resource demands and to maximize duplication within a training batch. RecD introduces a new tensor format, InverseKeyedJaggedTensors (IKJTs), to deduplicate feature values in each batch. We show how DLRM model architectures can leverage IKJTs to drastically increase training throughput. RecD improves the training and preprocessing throughput and storage efficiency by up to 2.48×, 1.79×, and 3.71×, respectively, in an industry-scale DLRM training system.
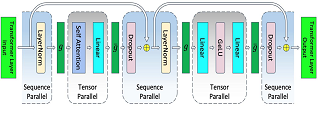
Abstract
Training large transformer models is one of the most important computational challenges of modern AI. In this paper, we show how to significantly accelerate the training of large transformer models by reducing activation recomputation. Activation recomputation is commonly used to work around memory capacity constraints. Rather than storing activations for backpropagation, they are traditionally recomputed, which saves memory but adds redundant compute. In this work, we show most of this redundant compute is unnecessary because we can reduce memory consumption sufficiently without it. We present two novel yet very simple techniques: sequence parallelism and selective activation recomputation. In conjunction with tensor parallelism, these techniques almost eliminate the need to recompute activations. We evaluate our approach on language models up to one trillion parameters in scale and show that our method reduces activation memory by 5x, while reducing execution time overhead from activation recomputation by over 90%. For example, when training a 530B parameter GPT-3 style model on 2240 NVIDIA A100 GPUs, we achieve a Model Flops Utilization of 54.2%, which is 29% faster than the 42.1% we achieve using recomputation.
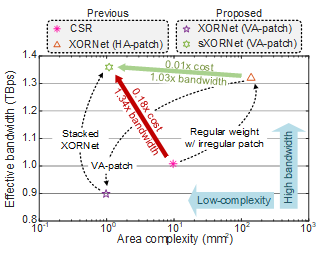
Abstract
This paper presents a new algorithm-hardware co-optimization approach that maximizes memory bandwidth utilization even for the pruned deep neural network (DNN) models.Targeting the well-known model compression approaches, for the first time, we carefully investigate the memory interface overheads caused by the irregular data accessing patterns.Then, the sparsity-aware memory interface architecture is newly developed to regularly access all the data of pruned-DNN models stored with the state-of-the-art XORNet compression.Moreover, we introduce the novel stacked XORNet solution for minimizing the number of data imbalances, remarkably relaxing the interface costs without slowing the effective memory bandwidth.As a result, experimental results show that our co-optimized interface architecture can achieve almost the ideal model-accessing speed with reasonable hardware overheads, successfully allowing the high-speed pruned-DNN inference scenarios.
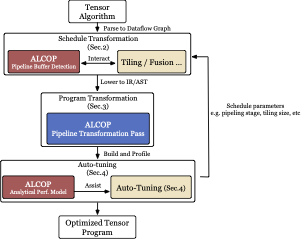
Abstract
Pipelining between data loading and computation is a critical tensor program optimization for GPUs. In order to unleash the high performance of latest GPUs, we must perform a synergetic optimization of multi-stage pipelining across the multi-level buffer hierarchy of GPU. Existing frameworks rely on hand-written libraries such as cuBLAS to perform pipelining optimization, which is inextensible to new operators and un-composable with prior tensor compiler optimizations. This paper presents ALCOP, the first framework that is compiler-native and fully supports multi-stage multi-level pipelining. ALCOP overcomes three critical obstacles in generating code for pipelining: detection of pipelining-applicable buffers, program transformation for multi-level multi-stage pipelining, and efficient schedule parameter search by incorporating static analysis. Experiments show that ALCOP can generate programs with 1.23× speedup on average (up to 1.73×) over vanilla TVM. On end-to-end models, ALCOP can improve upon TVM by up to 1.18×, and XLA by up to 1.64×. Besides, our performance model significantly improves the efficiency of the schedule tuning process and can find schedules with 99% of the performance given by exhaustive search while costing 40× fewer trials.
Abstract
In the domain of computer vision, transformer models have shown noteworthy success, prompting extensive research on optimizing their inference, particularly concerning their deployment on edge devices. While quantization has emerged as a viable solution for enabling energy efficiency in Convolutional Neural Networks (CNNs), achieving direct quantization of complex activation and normalization operators in transformer models proves to be a challenging task. Existing methods that rely on 64-bit integers often suffer from data truncation issues when deployed to energy-constrained edge devices, resulting in a significant loss of model accuracy. In this paper, we propose a range-constrained quantization technique for activation and normalization operators in transformers that addresses the dilemma between data range and precision. Our approach is the first 32-bit integer-based edge kernel implementation for vision transformers with post-training integer-only quantization, ensuring both efficiency and accuracy. Experimental results demonstrate a remarkable 5 times kernel speedup when deployed on two different ARM CPUs, with negligible accuracy loss in comparison to full-precision vision transformers. This innovative work is poised to significantly impact the deployment of transformer models on energy-efficient edge devices.
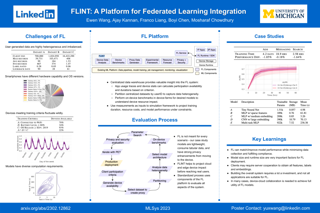
Abstract
Cross-device federated learning (FL) has been well-studied from algorithmic, system scalability, and training speed perspectives. Nonetheless, moving from centralized training to cross-device FL for millions or billions of devices presents many risks, including performance loss, developer inertia, poor user experience, and unexpected application failures. In addition, the corresponding infrastructure, development costs, and return on investment are difficult to estimate. In this paper, we present a device-cloud collaborative FL platform that integrates with an existing machine learning platform, providing tools to measure real-world constraints, assess infrastructure capabilities, evaluate model training performance, and estimate system resource requirements to responsibly bring FL into production. We also present a decision workflow that leverages the FL-integrated platform to comprehensively evaluate the trade-offs of cross-device FL and share our empirical evaluations of business-critical machine learning applications that impact hundreds of millions of users.
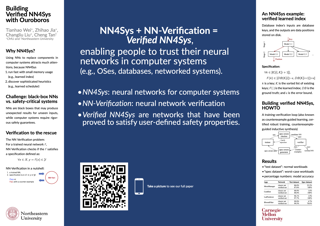
Abstract
Neural networks are powerful tools. Applying them in computer systems—operating systems, databases, and networked systems—attracts much attention. However, neural networks are complicated black boxes that may produce unexpected results. To train networks with well-defined behaviors, we introduce ouroboros, a system that constructs verified neural networks. Verified neural networks are those that satisfy user-defined safety properties, known as specifications. Ouroboros builds verified networks by a training-verification loop that combines deep learning training and neural network verification. The system employs multiple techniques to fill the gap between today’s verification and the properties required for systems. Ouroboros also accelerates the training-verification loop by spec-aware learning. Our experiments show that ouroboros can train verified networks for five applications that we study and has a 2.8× speedup on average compared with the vanilla training-verification loop.
Abstract
Data-parallel distributed training (DDT) is the de facto way to accelerate deep learning on multiple GPUs.In DDT, communication for gradient synchronization is the major efficiency bottleneck.Many gradient compression (GC) algorithms have been proposed to address this communication bottleneck by reducing the amount of communicated data.Unfortunately, it has been observed that GC only achieves moderate performance improvement in DDT, or even harms the performance.In this paper, we argue that the current way of deploying GC in a layer-wise fashion reduces communication time at the cost of non-negligible compression overheads.To address this problem, we propose Cupcake, a compression optimizer to fully unleash GC algorithms' advantages in accelerating DDT. It applies GC algorithms in a fusion fashion and determines the provably optimal fusion strategy to maximize the training throughput of compression-enabled DDT jobs.Experimental evaluations show that GC algorithms with Cupcake can achieve up to 2.03x speedup in training throughput over training without GC, and up to 1.79x speedup over the state-of-the-art approaches of applying GC to DDT in a layer-wise fashion.
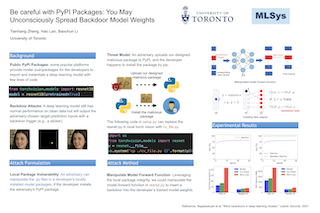
Abstract
To facilitate deep learning project development, some popular platforms provide model (sub)packages for developers to import and instantiate a deep learning model with few lines of code. For example, PyTorch provides \texttt{torchvision.models} for developers to instantiate models such as VGG and ResNet. Although those model packages are easy to install and use, their integrity may not be well-protected locally. In this paper, we show that an adversary can manipulate the \texttt{.py} files in the developers' locally installed model packages, if the developers install the adversary's PyPI package for using its claimed features. When installing the adversary's package, the system does not report any warning or error related to the manipulation. Leveraging this integrity vulnerability, we design an attack to manipulate the model forward function in the local \texttt{.py} files, such as \texttt{resnet.py} in the local \texttt{torchvision.models} subpackage. With our attack, the adversary can implant a backdoor into the developers' trained model weights, even supposing that the developers use seemingly clean training data and seemingly normal training code.
Abstract
Pipeline parallelism enables efficient training of Large Language Models (LLMs) on large-scale distributed accelerator clusters. Yet, pipeline bubbles during startup and tear-down reduce the utilization of accelerators. Although efficient pipeline schemes with micro-batching and bidirectional pipelines have been proposed to maximize utilization, a significant number of bubbles cannot be filled using synchronous forward and backward passes. To address this problem, we suggest that extra work be assigned to the bubbles to gain auxiliary benefits in LLM training. As an example in this direction, we propose PipeFisher, which assigns the work of K-FAC, a second-order optimization method based on the Fisher information matrix, to the bubbles to accelerate convergence. In Phase 1 pretraining of BERT-Base and -Large models, PipeFisher reduces the (simulated) training time to 50-75% compared to training with a first-order optimizer by greatly improving the accelerator utilization and benefiting from the improved convergence by K-FAC.
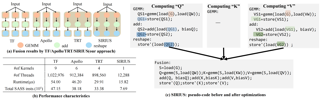
Abstract
As emerging applications are rapidly moving to accelerators, a greatdeal of research has been proposed to improve the performance of the accelerators. For the AI applications, fruitful software-driven research has been focused on proposing new programming languages, new kernel fusion heuristics,new optimization tuning approaches, and new software execution engines. However, how to leverage classical compiler optimizations to generate efficient code is an overlooked aspect of performance. In this paper, we propose a whole-program analysis and optimization compiler framework, SIRIUS, to uniformly model the host and kernel computations in a unified polyhedral representation and,further, seek maximal fusion opportunities from the global view so that the fused kernel can benefit from classical optimizations. Evaluations over representative DNN models demonstrate that SIRIUS can achieve up to 11.98x speedup over TensorRT, and 154.84x speedup over TensorFlow. In particular, for BERT, SIRIUS can achieve 1.46x speedup over TensorRT.
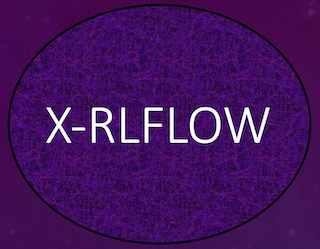
Abstract
Tensor graph superoptimisation systems perform a sequence of subgraph substitution to neural networks, to find the optimal computation graph structure. Such a graph transformation process naturally falls into the framework of sequential decision-making, and existing systems typically employ a greedy search approach, which cannot explore the whole search space as it cannot tolerate a temporary loss of performance. In this paper, we address the tensor graph superoptimisation problem by exploring an alternative search approach, reinforcement learning (RL). Our proposed approach, X-RLflow, can learn to perform neural network dataflow graph rewriting, which substitutes a subgraph one at a time. X-RLflow is based on a model-free RL agent that uses a graph neural network (GNN) to encode the target computation graph and outputs a transformed computation graph iteratively. We show that our approach can outperform state-of-the-art superoptimisation systems over a range of deep learning models and achieve by up to 40% on those that are based on transformer-style architectures. We show that our approach can outperform state-of-the-art superoptimisation systems over a range of deep learning models and achieve by up to 40% on those that are based on transformer-style architectures.
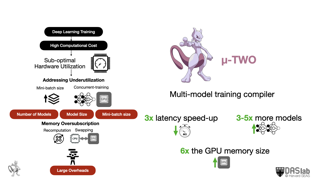
Abstract
In this paper, we identify that modern GPUs - the key platform for developing neural networks - are being severely underutilized, with ∼ 50% utilization, that further drops as GPUs get faster. We show that state-of-the-art training techniques that employ operator fusion and larger mini-batch size to improve GPU utilization are limited by memory and do not scale with the size and number of models. Additionally, we show that using state-of-the art data swapping techniques (between GPU and host memory) to address GPU memory limitations lead to massive computation stalls as network sizes grow.We introduce μ-two, a novel compiler that maximizes GPU utilization. At the core of μ-two is an approach that leverages selective data swapping from GPU to host memory only when absolutely necessary, and maximally overlaps data movement with independent computation operations such that GPUs never have to wait for data. By collecting accurate run-time statistics and data dependencies, μ-two automatically fuses operators across different models, and precisely schedules data movement and computation operations to enable concurrent training of multiple models with minimum stall time. We show how to generate μ-two schedules for diverse neural network and GPU architectures and integrate μ-two into the PyTorch framework. Our experiments …
Abstract
Distributed training technologies have advanced rapidly in the past few years and have unlocked unprecedented scalability with increasingly complex solutions. These technologies have made distributed training much more efficient and accessible, though they impose specific constraints on the training paradigm or the model structure. As a result, applications that fail to meet these constraints must rely on general-purpose distributed computing frameworks to scale out. However, without access to the internal components of deep learning frameworks, these distributed computing frameworks usually significantly fall short in terms of efficiency and usability. To address these problems, we propose PyTorch RPC as a generic and high-performance solution for distributed deep learning. Compared to generic distributed computing frameworks, PyTorch RPC natively provides essential features for implementing training applications in a distributed environment, including optimized tensor communications, remote memory management, and distributed autograd. Evaluations show that PyTorch RPC attains up to two orders of magnitude faster tensor communication compared to gRPC with one-tenth of the user code. Case studies further demonstrate that users can easily employ PyTorch RPC to build efficient reinforcement learning applications (video game solver), implement large language models (GPT3), train recommendation models (DLRM), and scale federated learning tasks (FedML).
Abstract
Recent research has shown that training low-rank neural networks can effectively reduce the total number of trainable parameters without sacrificing predictive accuracy, resulting in end-to-end speedups. However, low-rank model training necessitates adjusting several additional factorization hyperparameters, such as the rank of the factorization at each layer. In this paper, we tackle this challenge by introducing Cuttlefish, an automated low-rank training approach that eliminates the need for tuning factorization hyperparameters. Cuttlefish leverages the observation that after a few epochs of full-rank training, the stable rank (i.e., an approximation of the true rank) of each layer stabilizes at a constant value. Cuttlefish switches from full-rank to low-rank training once the stable ranks of all layers have converged, setting the dimension of each factorization to its corresponding stable rank. Our results show that Cuttlefish generates models up to 5.6 times smaller than full-rank models, and attains up to a 1.2 times faster end-to-end training process while preserving comparable accuracy. Moreover, Cuttlefish outperforms state-of-the-art low-rank model training methods and other prominent baselines. The source code for our implementation can be found at: https://github.com/hwang595/Cuttlefish.
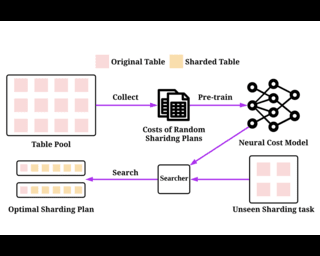
Abstract
Sharding a large machine learning model across multiple devices to balance the costs is important in distributed training. This is challenging because partitioning is NP-hard, and estimating the costs accurately and efficiently is difficult. In this work, we explore a "pre-train, and search" paradigm for efficient sharding. The idea is to pre-train a universal and once-for-all neural network to predict the costs of all the possible shards, which serves as an efficient sharding simulator. Built upon this pre-trained cost model, we then perform an online search to identify the best sharding plans given any specific sharding task. We instantiate this idea in deep learning recommendation models (DLRMs) and propose NeuroShard for embedding table sharding. NeuroShard pre-trains neural cost models on augmented tables to cover various sharding scenarios. Then it identifies the best column-wise and table-wise sharding plans with beam search and greedy grid search, respectively. Experiments show that NeuroShard significantly and consistently outperforms the state-of-the-art on the benchmark sharding dataset, achieving up to 23.8% improvement. When deployed in an ultra-large production DLRM with multi-terabyte embedding tables, NeuroShard achieves 11.6% improvement in embedding costs over the state-of-the-art, which translates to 6.6% end-to-end training throughput improvement. To facilitate future research of the …
Abstract
We present MegaBlocks, a system for efficient Mixture-of-Experts (MoE) training on GPUs. Our system ismotivated by the limitations of current frameworks, which restrict the dynamic routing in MoE layers to satisfythe constraints of existing software and hardware. These formulations force a tradeoff between model quality andhardware efficiency, as users must choose between dropping tokens from the computation or wasting computationand memory on padding. To address these limitations, we reformulate MoE computation in terms of block-sparseoperations and develop new block-sparse GPU kernels that efficiently handle the dynamism present in MoEs. Ourapproach never drops tokens and maps efficiently to modern hardware, enabling end-to-end training speedups ofup to 40% over MoEs trained with the state-of-the-art Tutel library and 2.4× over dense DNNs trained with thehighly-optimized Megatron-LM framework.